18 April 2016 to 22 April 2016
Mathematik-Zentrum, Lipschitz Lecture Hall, Endenicher Allee 60, Bonn
Organizers: André Uschmajew (Universität Bonn)
Description: Low-rank tensor approximation is an established tool in signal processing and data analysis to decompose multilinear data beyond standard matrix principal component analysis. In recent years its application to modern large scale problems has become an active area of research. A different motivation for the development of low-rank tensor techniques comes from the "curse of dimensionality” after discretization of high-dimensional functions as they arise, for example, in quantum physics or uncertainty quantification. When the number of variables becomes unmanagebly large, traditional numerical methods for solving (partial) differential, integral, or eigenvalue equations are severely limited in their application.
The transition from low-rank matrix to low-rank tensor approximation involves many surprisingly hard challenges and open problems on the theoretical and practical level. To investigate these problems, it is important to combine the developments from different fields within numerical mathematics and optimization that aim at understanding and developing low-rank tensor techniques. In particular, there is a fruitful interaction of theoretical tools from different mathematical branches, such as approximation theory, algebraic/differential geometry, linear algebra, and numerical analysis, on the one side, and there are various application areas in big data, quantum physics, computational chemistry, or computer science, on the other side.
This Hausdorff School is intended for graduate and postdoctoral students who are interested in classical results and recent developments in low-rank tensor approximation, and wish to acquire modern research tools to work in the field. Particular focus will be on high-dimensional numerical tensor calculus, and low-rank optimization methods.
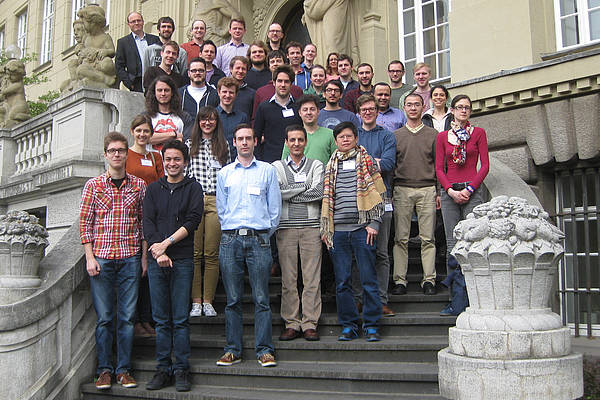
Lecture Series by:
- Lars Grasedyck (RWTH Aachen)
- Hierarchical Tucker format
- Blackbox / Cross approximation
- Outlook, applications and discussion
- Bart Vandereycken (Université de Genève)
- Tensor trains and matrix product states
- Riemannian optimization for tensors
- Quantized tensor trains
- André Uschmajew (Universität Bonn)
- Tensor product of Hilbert spaces
- Low-rank approximability
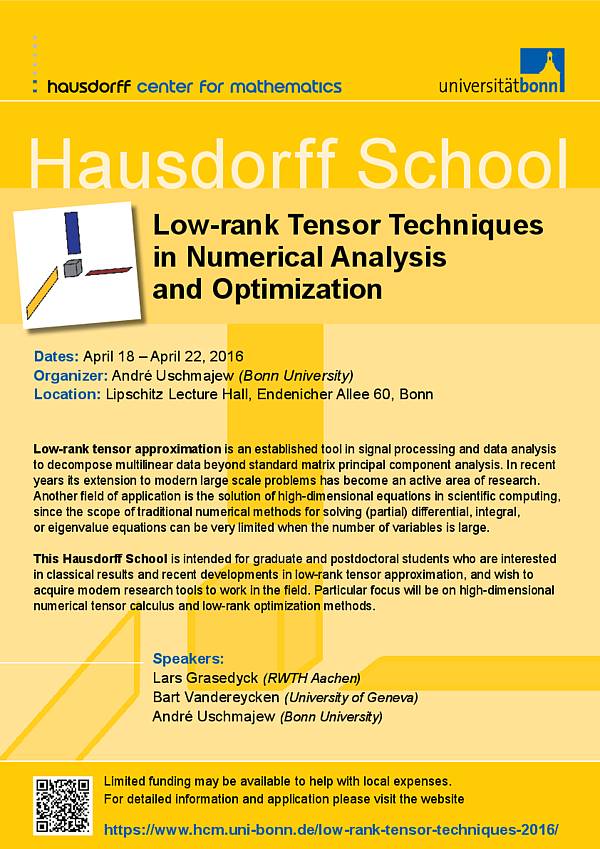
Person |
Affiliation |
Period of stay |
Moritz August | Technical University of Munich | |
Oleg Balabanov | Ecole Centrale de Nantes | |
Martijn Boussé | KU Leuven | |
Daan Camps | KU Leuven | |
Otto Debals | KU Leuven | |
Reinier Doelman | TU Delft | |
Simon Etter | University of Warwick | |
Lars Grasedyck | RWTH Aachen | |
Behnam Hashemi | Mathematical Institute, University of Oxford | |
Benjamin Huber | Technische Universitaet Berlin | |
Ben Jeuris | KU Leuven | |
René Kehl | TU Berlin | |
Kristin Kirchner | Chalmers University of Technology | |
Erna Begovic Kovac | University of Zagreb | |
Benjamin Kutschan | TU Berlin | |
Maxim Kuznetsov | Lomonosov Moscow State University | |
Christian Kümmerle | Technische Universität München | |
Dana Lahat | GIPSA-Lab | |
Alexander Litvinenko | KAUST | |
Dimitrios Loukrezis | TU Darmstadt | |
Johannes Maly | TU München | |
Davide Palitta | Università di Bologna | |
Lana Perisa | University of Split, Croatia | |
Max Pfeffer | Technische Universitaet Berlin | |
Duong Luu Trung Pham | University of Luxembourg | |
Luis Garcia Ramos | Technische Univiersitaet Berlin | |
Rishi Relan Vrije | Universiteit Brussel | |
Emil Ringh | KTH Royal Institute of Technology | |
Erik Marc Schetzke | Universität Oldenburg | |
Carlos Echeverria Serur | Technische Universität Berlin | |
Baptiste Sinquin | TU Delft | |
Bedrich Sousedik | University of Maryland, Baltimore County | |
André Uschmajew | Universität Bonn | |
Bart Vandereycken | University of Geneva | |
Hanna Walach | Tuebingen University | |
Sebastian Wolf | Technische Universität Berlin | |
Chengpu Yu | Delft University of Technology |